What makes clinical machine learning fair?
In their new paper, lab members Effy Vayena and Alessandro Blasimme and co-authors Marine Hoche, Olga Mineeva, and Gunnar Rätsch investigate this question.
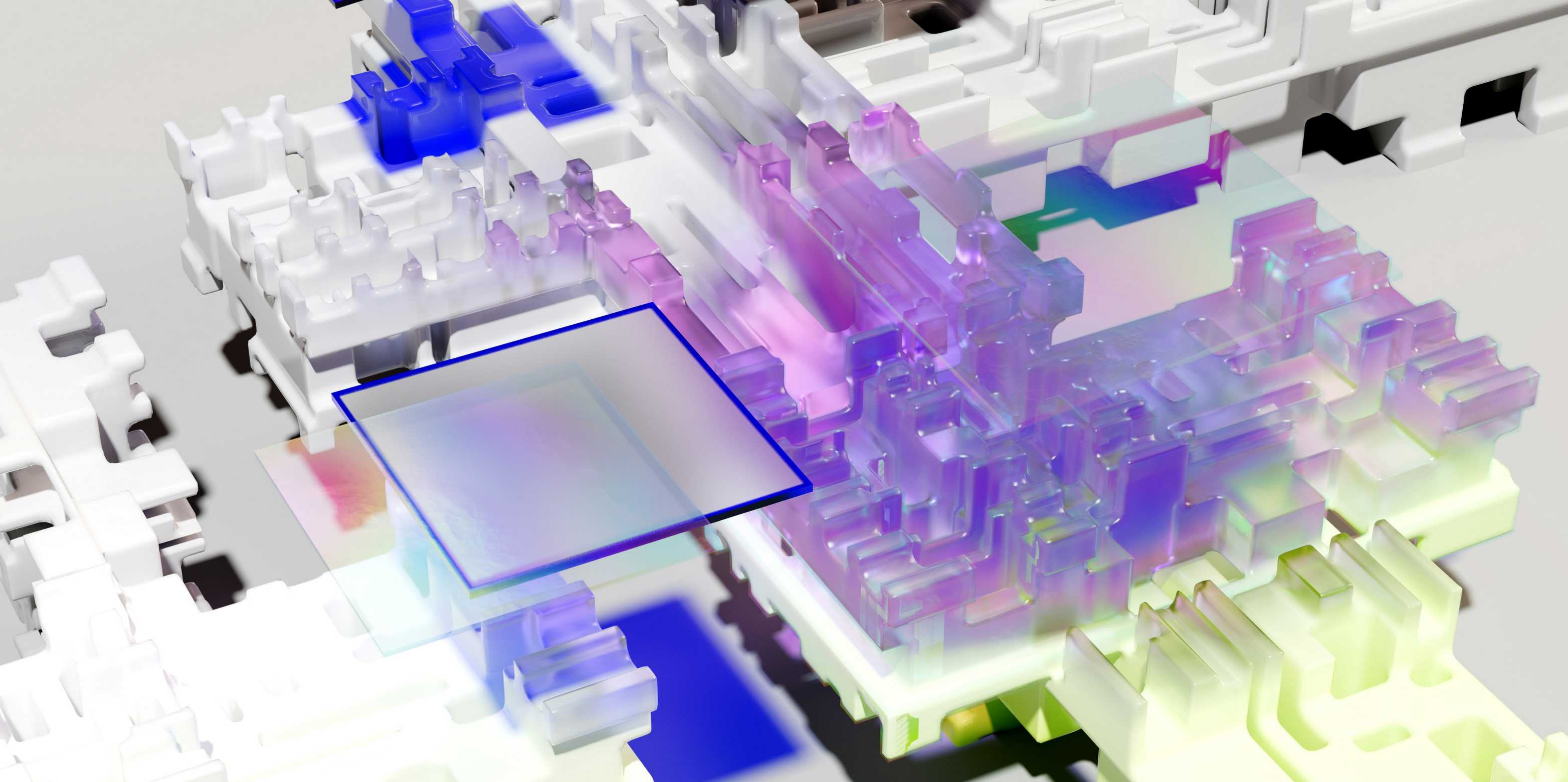
The allure of machine learning (ML) in medicine is strong. The prospect of optimizing clinical decision-making, reducing medical errors, boosting diagnostic accuracy, and achieving superior patient outcomes is undeniably attractive. This potential for a paradigm shift through the widespread and rapid incorporation of ML into clinical workflows has generated significant excitement.
However, this very enthusiasm, this optimistic vision of an AI-powered future for medicine, is tempered by crucial ethical considerations. And among these, one issue looms particularly large, casting a long shadow over the promise: the pervasive challenge of algorithmic bias. This inherent risk, capable of subtly yet profoundly skewing the outputs of even the most sophisticated ML models, demands our unwavering attention as we navigate this transformative era.
Recognizing this critical juncture, in this new paper, the authors introduce a practical framework that integrates ethical and technical requirements for assessing and minimizing AI bias in clinical practice. This operationalizable framework aims to “cultivate fairness, transparency and enhanced healthcare outcomes in the realm of clinical ML.”
Read the article in full:
Hoche, M., Mineeva, O., Rätsch, G., Vayena, E., & Blasimme, A. (2025). What makes clinical machine learning fair? A practical ethics framework. PLOS Digital Health, 4(3), e0000728. external page https://doi.org/10.1371/journal.pdig.0000728